Two handed touchy-feely robot can pick a peck of pickled Pringles
10 Sep 2023
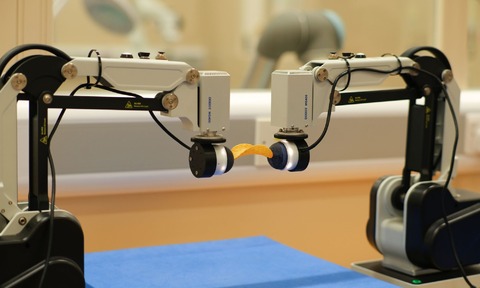
Scientists at the University of Bristol say their AI enhanced, bi-manual robot has achieved ‘near-human’ levels of sensitivity that allow it to pluck a single crisp without breaking it.
Their Bi-Touch system, housed at the Bristol Robotics Laboratory, relies upon deciphering instructions from a digital assistant which they suggest could revolutionise industries such as crop picking, domestic service, and eventually recreate touch in artificial limbs
Published in the Institute of Electrical and Electronics Engineers’ IEEE Robotics and Automation Letters, the team’s research explains how AI agents perceive their surroundings through tactile and proprioceptive feedback, so guiding the robot's actions.
Achieving bimanual manipulation with tactile feedback is a critical milestone in attaining human-level robot dexterity.
Lead author Yijiong Lin from Bristol’s Faculty of Engineering explained the potential for industrial and other settings:
"With our Bi-Touch system, we can easily train AI agents in a virtual world within a couple of hours to achieve bimanual tasks that are tailored towards the sense of touch.
“More importantly, we can directly apply these trained agents from the virtual world to the real world without further training. The tactile bimanual agent can perform tasks even in the face of unexpected disturbances and handle delicate objects gently."
The complexity of designing the necessary controllers and the availability of suitable hardware has until now meant that bi-manual research has been less explored compared to single-arm robotics. Recent advances in AI and robotic tactile sensing contributed to the reseacrhers success.
Scientists created a virtual simulation with two robot arms equipped with tactile sensors, using a goal-update mechanism to give robot agents the incentive to learn bi-manual tasks. The researchers then transferred this knowledge to an actual tactile dual-arm robot system, demonstrating the practical applicability of their approach.
The robot learns bimanual skills through Deep Reinforcement Learning (Deep-RL), allowing it to learn through trial and error. The robot makes decisions by attempting various behaviours to achieve designated tasks, such as lifting objects without dropping or damaging them. Successful attempts are rewarded, while failures inform the robot about what not to do.
The AI agent relies solely on proprioceptive feedback— the ability to sense movement, action, and location—and tactile feedbackind. It was able to safely lift extremely fragile items, including a fragile object in the shape of a single Pringle crisp.
Co-author Professor Nathan Lepora stated: "Our Bi-Touch system presents a promising approach with cost-effective software and hardware for teaching bimanual behaviors with a sense of touch in simulation, which can be directly applied in the real world. The open source nature of our tactile dual-arm robot simulation allows for further research into a variety of tasks."
In conclusion, Yijiong Lin added that it enabled a tactile dual-arm robot to learn exclusively from simulation and accomplish various manipulation tasks gently in the real world, saying it was now possible to train AI agents in a virtual world within a few hours to perform bimanual tasks tailored to the sense of touch.
Pic: Yijiong Li