How holistic analytics saved a company from losing business worth millions
20 Jun 2018
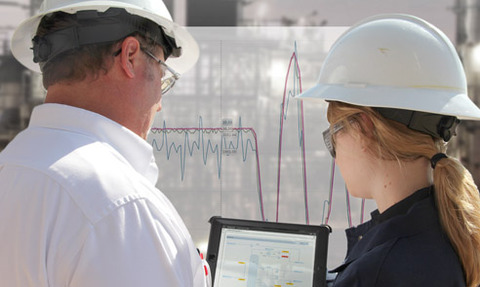
Honeywell expertise helped save one client business worth $20 million a year by rapidly identifying the cause of a chemical quality issue for a supplier.
A silicon wafer manufacturer discovered that one of the chemical products supplied to it experienced an unexplained step change in a quality variable.
The deviation affected all of its factories starting with material manufactured on a particular date. As a result it had begun to fail quality tests, putting at risk the equivalent of more than £14 million a year in future business.
Honeywell Electronic Materials (HEM), which provided the product and has supplied critical materials to the semiconductor industry for more than half a century, was keen to identify the root cause .
HEM records a wide range of process data as standard practice, including temperature, ambient conditions, raw material suppliers and composition, and quality data from multiple customers. It was therefore able to explore the historical data to try to discover and quantify contributing factors.
Expertise and analytical support confirmed no change had occurred to the chemical composition of the product and thus no contaminate existed
Initial investigation by the HEM team narrowed the likely root cause down to a few key environmental and process variables, yet a definitive correlation to the quality issue remained elusive. Moreover, some data was recorded only on paper, limiting the data fidelity and reducing opportunities to include this data in computer analysis.
HEM’s sister company, Honeywell Process Solutions (HPS), assisted. Its Connected Plant group used Sentience Data Analytics to help with data analysis and to identify the cause of the property change.
In parallel, a Honeywell corporate R&D team provided expertise and analytical support that confirmed no change had occurred to the chemical composition of the product and thus no contaminate existed.
Multi-team approach
The Honeywell Connected Plant data analytics team worked to obtain the necessary data, perform exploratory analyses, and quantitatively model the impact of contributing factors before suggesting corrective actions. Root cause analysis determined which process variables to monitor, fix, or automate with closed- loop control.
The joint data analytics and subject matter expert team identified factory temperature and humidity during production as potentially playing a role in the quality change observed. The team digitised the existing hard copies by scanning them and then used image analysis to convert the temperature and humidity readings into electronic data.
They employed Honeywell Uniformance® Suite, which offers a range of data analytics software. Several machine learning techniques were employed for the analysis, including regression, SVM, random forest and decision trees.
Causes isolated
Analysis revealed two key factors were largely responsible for the change in the chemicals supplied:
- Environmental factors and, in particular, the factory temperature and relative humidity were the most significant factors correlated with the deviating variable and with one another
- Reaction temperature and times during the process were also a factor, and analysis narrowed the lead contributors down to the warm up process
These issues could be addressed through new process controls: temperature control in the factory and tighter process control on the reaction. This helped reduce manufacturing variability and thus improved quality.
Honeywell Connected Plant data analytics employed to identify key contributing factors to process variability was instrumental to the whole team effort that demonstrated no other change had occurred to the chemistry.
Combining Honeywell Connected Plant data analytics expertise and Uniformance analysis with HEM’s in-depth process ?knowledge, HEM was able to identify and resolve an issue presenting a real risk to customer satisfaction, production costs and ongoing revenues. It also ensured the project delivered a rapid and substantial ROI.