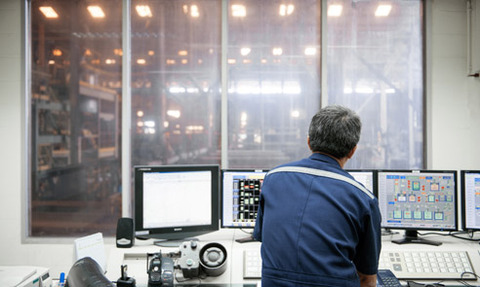
To harness the best from predictive maintenance tools and processes, there is no escaping the need to embrace 4.0 – otherwise the data, analytics and insights aren’t at your disposal, explains Michelle Knott.
Shifting from a programme of planned maintenance to one of predictive maintenance can be game-changing for process operators. The benefit most frequently cited is drastically reduced downtime, but other advantages such as elimination of over-maintenance, better control of spares stocks and increased transparency are all probable consequences of the ability to spot when and where future breakdowns are going to occur, so they can be fixed before they disrupt operations.
“Driven on by improvements in efficiency, reductions in costly downtime, greater flexibility and more powerful insights and visibility of what is happening in their business, the uptake of data reliant technologies and infrastructure will continue to grow quickly in the age of the fourth industrial revolution,” says Greg Hookings, head of business development – digitalisation, with edge computing specialist, Stratus Technologies.
Everyone agrees that data holds the key to unlocking this bright new future. The arrival of algorithms that enable artificial intelligence and machine learning is now enabling condition monitoring systems to observe and understand enough about an asset to detect nascent problems. Instead of waiting for an experienced operator to notice motors making a funny noise, for example, such systems can identify the problem before it impacts on operations.
Modern systems can be implemented and maintained by OT [operational technology] staff, reducing the burden on IT teams and associated skills
Greg Hookings, head of business development – digitalisation, Stratus Technologies
“This style of predictive maintenance relies on complete, plantwide data collection and monitoring, in combination with intimate knowledge of equipment parameters, to spot warning signs and set flags well before the equipment exhibits issues,” says George Walker, managing director of Novotek UK & Ireland. Novotek offers GE Digital’s Historian software, which is one of the new generation of solutions aiming to harness the power of data in this way.
The Senseye PdM solution is another example. It uses predictive analytics to deliver a forecast of the remaining useful life (RUL) of many assets. As well as enabling operators to run industrial processes more efficiently, Senseye argues that this key insight offers great potential to transform the way asset owners and their suppliers do business. With the RUL at their fingertips, operators and original equipment manufacturers (OEMs) can plan when to repair or replace an asset in order to optimise budgets, resources and processes.
It can even impact on contractual arrangements. Process operators might no longer buy an asset as such, and instead buy the function that the asset delivers. It’s a set-up known as ‘servitisation’.
“Servitisation means committing to deliver a function, rather than simply providing assets. So a robotics supplier might commit to delivering a certain number of welds per hour for a car maker, or an aircraft supplier might promise an airline a certain number of flying hours per month,” explains Robert Russell, chief technology officer with Senseye.
The same principle might apply just as well to process equipment ranging from bottling machines to metal detectors, or from pumps to multi-head weighers. But the risk associated with providing the right level of support has previously deterred many OEMs from adopting the servitisation model.
“It’s a contractual model that has already taken off in the high-value, high-stakes arena of defence and aerospace, where so called integrated operational support (IOS) agreements are now the norm,” says Russell.
“But servitisation hasn’t attracted the same level of interest in many other industries before now. One reason is that the potential benefits of servitisation also come with risks for OEMs. If they commit to delivering a certain level of service, they’re also signing up to potential penalties if things go wrong. In other words, they’re shouldering a risk that would previously have been borne by their customer, the end user.”
He argues that affordable solutions for condition monitoring now mitigate that risk by enabling true predictive maintenance, making the shift to servitisation an appealing prospect for process operators and OEMs alike. “Predictive maintenance is the critical difference and condition monitoring provides the tools that enable it,” he confirms.
The potential benefits of servitisation also come with risks for OEMs. If they commit to delivering a certain level of service, they’re also signing up to potential penalties if things go wrong
Robert Russell, chief technology officer, Senseye
With the need for OEMs to monitor their equipment remotely, servitisation lends itself to Cloud-based condition monitoring /predictive maintenance solutions. Cloud-based solutions also offer advantages in terms of scalability, which helps keep costs under control and minimises any upfront risks for the process operator. This is regardless of the number of assets they are including in the implementation.
However, the Cloud provides no silver bullet. Having some of the computing power needed to implement condition monitoring situated closer to the assets (at the so-called ‘edge’) can offer its own advantages. “There is no ‘one size fits all approach’ to the distribution of computing but understanding the advantages to different methods helps to unlock the huge potential benefits,” says Hookings.
“Starting at the device level, there are numerous advantages to be gained from an edge computing platform. The latest edge platforms are built to be rugged enough to operate in increasingly harsh environments and to the very high levels of uptime required at the application level. Computing at this level provides significant other benefits too – modern systems can be implemented and maintained by OT [operational technology] staff, reducing the burden on IT teams and associated skills.”
“With built in security, such systems also offer some peace of mind to organisations rightly concerned about cyber-security. In addition, managing data on site and not sending it to the Ccloud offers an inherent security benefit as critical data is not transferred over the internet.”
Depending on how responsive any feedback needs to be, reduced latency can also be an advantage of retaining some computing power close to home.
“Latency is hugely reduced when compared with managing data remotely. This opens the door to real-time operator visibility of the system and information that can inform real-time decisions at the device level,” says Hookings [pictured]. “With predictive and preventative maintenance trends offering significant return on investment, edge level computing is ideally placed to feature as an increasingly important part of industry 4.0 strategies.”
“Moving up from the device level to the server room allows enterprises to manage larger amounts of data and bring in aspects of supply chain management, overall energy consumption and other business level data.”
But he acknowledges the contrasting benefits of Cloud computing, including the ability to handle very big data sets. Most firms can adopt a hybrid with elements of edge and Cloud computing.
There are a number of alternatives when it comes to implementing predictive maintenance but two essential prerequisites behind any successful implementation: deploying the right instrumentation to capture the right data and a smart solution that can use that information to generate insights. That may sound obvious, but Russell says many companies remain unsure about how to leverage the data they are already collecting to deliver more value.
“For, the idea of leveraging artificial intelligence can be a daunting prospect. However, it’s not an ‘all or nothing’ deal. In fact, it’s quite common to find that some parts of an organisation are better prepared than others to make a start,” he says. In other words, data is often being collected already that can be brought together to deliver key insights. Russell explains Senseye sometimes implements its solution across as few as 50 assets before scaling up: “This makes it easier to justify investment of time and effort that can bring other areas of the organisation to a level of preparedness that enables them to benefit.”
Senseye says its own solution typically starts producing insights after around 24 days and customers usually achieve ROI within three months.