Think of AI as investment in productivity and efficiency rather than cost
27 Oct 2021
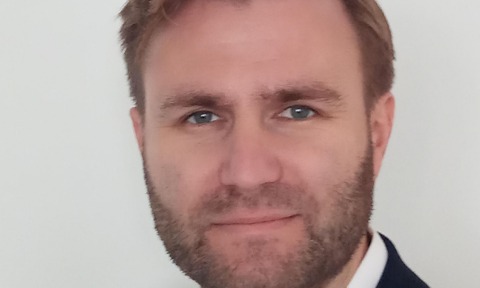
Concerns about AI’s investment should be offset benefits in terms of efficiency, productivity and costs says Encora Energy’s Andrew Normand...
Equipment readings can be impacted by a range of different factors based on conditions upstream or downstream, through auxiliary equipment and environmental factors such as sudden, unexpected deviations in weather patterns.
Plant operators have to settle for identifying more pronounced symptoms with limited signals to avoid false alarms, whilst still catching an issue before a major failure. However, this doesn’t identify the upstream/downstream operations that may have caused the issue.
This can lead to numerous problems including system failure, loss of efficiency and reduced availability leading to lost productivity – which could potentially cost plant operators millions of pounds in lost revenue. Therefore, there’s a need for a technology that can review the entire system as a whole, taking in more than just the main equipment but also the environmental factors and the impacts of auxiliary systems and operating conditions.
AI intelligence can remove the affecting variables to reveal the underlying performance of a system. New patterns that weren’t previously visible can now be identified.
UptimeAI’s ‘AI Expert’ software, for example, uses an AI engine that continuously learns from historic and ongoing data and identifies how each of the parameters involved in the system change in relation to each other. From this data, it is able to continually read current new data and predict an expected value based on other parameters. It then compares this predicted value against the actual data and determines any discrepancies, creating an anomaly score that indicates the overall health of the system.
It also runs a diagnostic engine with built-in world-class domain knowledge to diagnose and define the issue. It’s also capable of continuously learning from new experiences and can recognise new types of events – effectively learning in the same way that a human engineer learns.
UptimeAI was asked to look at the efficiency of a condensing steam turbine in a power plant to determine any lost efficiency and improvements that could be made.
Software was fed with historic data for the entire turbine system which included not just the turbine itself, but also the condenser and the entire cooling water circuit as one system. The turbine system was heavily influenced by a large variance in cooling water temperature due to seasonal and daily environmental changes.
Feeding historic data through the AI application revealed a continued upward trend including three targeted alarms over 36 months indicating points of significant change.
The alarms were generated taking into account the impacts of turbine exhaust, seasonality and load fluctuations. By analysing where the anomalies were greatest, the AI diagnosis tool was able to make predictions on the likely failure mechanisms and prescriptive recommendations using the application’s built-in engineering knowledge. This was able to diagnose specific cooling water issues that were affecting the efficiency of the condenser and hence the turbine.
The UptimeAI application was able to identify a total improvement opportunity of 0.016bar of condenser vacuum. This was made up of cooling water tower/return inefficiency (circa 25%), cooling water discharge pump low pressure (circa 24%), condenser fouling (circa 32%) and air ingress (circa 19%). This equated to a 2.2% improvement opportunity in backpressure worth an estimated £140,000 per year in increased efficiency.
Andrew Normand is UptimeAI partnership lead for Encora Energy