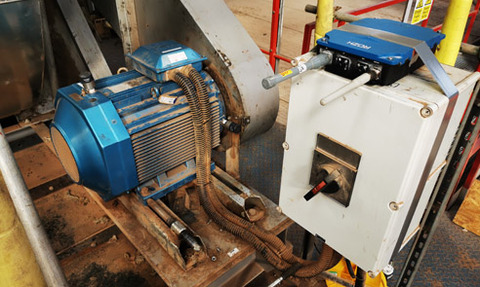
Condition monitoring has moved light years from the ‘see, hear’ days with first automation and now intelligent systems doing the really heavy lifting.
“In the 1970s and 80s, condition monitoring was largely restricted to audible monitoring with technology playing little part. In other words, you literally listened to the equipment.”
So explains Jason Tweedy, head of condition monitoring at Brammer Buck & Hickman, adding that, similarly, a visual inspection could also provide key information about problems leading to wear.
“Of course, it’s not always possible to see all parts on a machine or hear every nuance and there’s not always a person on site who has worked with that specific machinery for years and can tell when something isn’t quite right. Plus, by the time you can hear or see something isn’t right, the damage has often already been done.”
Yet while humans have their pronounced limitations, thanks to automation and digitalisation, the boundaries of condition monitoring have expanded incrementally in recent decades.
New patterns that weren’t previously visible can now be identified. Problems can be detected much easier and be rectified before they cause issues
Andrew Normand, UptimeAI partnership lead, Encora Energy
For Peacock Engineering co-founder Alan Cambridge and UptimeAI partnership lead for Encora Energy Andrew Normand, the future of asset management lies in the exploitation of artificial intelligence.
Explains Normand: “Where real-time plant monitoring was previously used primarily to create reliability alarms on individual critical items of rotating equipment, the latest generation of AI is capable of full plant monitoring, detecting and diagnosing efficiency issues and data anomalies over entire process systems – a valuable commodity for time-pressed process engineers.”
In the last few years, he says, continuous monitoring of process plants has evolved dramatically with the move to continuous learning systems that will detect anomalies previously hidden under a sea of noise and provide diagnosis of specific issues in real time.
Previously, notes Cambridge, AI development was aimed primarily at quality control – spotting issues on production lines for early intervention, thus reducing downtime. This is ideal in a manufacturing environment, he continues, where a constant stream of data, and uniform assets with very few external variables, enable rapid machine learning.
“But it depends on errors being made in the production process – which may be common and acceptable in a manufacturing situation but is more problematic in other industries where assets may be varied, and corresponding data sets required to train algorithms are vast and not developed.”
Behaviour pattern
Modern AI offers the ability to reviewing the entire system holistically – not only critical equipment but also, says Normand, “the interrelations across the plant, environmental factors and the impacts of auxiliary systems and operating conditions”.
“This intelligence can remove the affecting variables and identify anomalous data for correction, revealing the underlying performance of a system. New patterns that weren’t previously visible can now be identified. Problems can be detected much easier and be rectified before they cause issues.”
So far, so good in principle. But the reality, as both experts admit, is that process and other businesses have a complex legacy of assets and priorities, as well as financial limitations that might inhibit their ability to adopt the best condition monitoring tools in practice.
Heavily regulated businesses cannot afford to have errors in their processes, so a huge part of their EAM effort is geared towards standardising processes to eliminate any possibility of errors. This may, acknowledges Cambridge, result in over-maintenance because there is no alternative to ensure uninterrupted service in all circumstances. Also, the vast number of variables in their assets makes it extremely difficult to collect the data necessary to train AI algorithms.
Normand concurs, referencing the growing focus on energy use as a component of process plant operations: improving energy efficiency or monitoring greenhouse gas emissions, it has become critical for plant viability.
“The search to improve energy use has resulted in engineers spending significant effort to monitor plant, understand where gains can be made, diagnose the reasons for inefficiency and finally to make improvements.”
This demand has produced a range of different tools and methods to capture, verify and analyse energy data but can, cautions Normand, give rise to ‘spreadsheet-hell’, where continuous maintenance and correction detracts from actually making improvements.
Laying the groundwork for transition to more sophisticated AI-based condition monitoring is key, suggests Cambridge, notably mobile solutions presented by SCADA sensors: “The extra data mobile solutions collect will help you to implement AI-based solutions sooner. Even if it seems there is no immediate application for AI-based maintenance in your business, having the additional data will make it easier to implement when more AI-based solutions emerge.”
Thus, field technicians equipped with mobile devices can fully access the AM system to gain instant notifications from sensor alarms, while scheduling and workflow systems within the EAM can alert the best qualified or nearest engineers to attend the issue.
Even if it seems there is no immediate application for AI-based maintenance in your business, having the additional data will make it easier to implement when more AI-based solutions emerge
Alan Cambridge, co-founder, Peacock Engineering
An AI application that can be set up easily and provide proper diagnosis of issues and boasts continuous self-learning to eliminate time-consuming maintenance can also diagnose reliability issues, adds Normand.
This provides a multi-disciplinary view to plant monitoring and benefits across multiple plant teams, minimising downtime and improving plant efficiency. There’s a human benefit too: “A good AI application increases insight but also reduces analytical workload, freeing up engineers to find the practical solutions to problems – problems that AI on its own is unable to solve.”
Alongside the holistic approach offered by AI, specific condition monitoring techniques that have been enhanced through technological advances are receiving renewed attention. Among these are improved forms of vibration analysis, suggests Brammer Buck & Hickman’s Jason Tweedy.
That trusted method in the process armoury, visual inspection, can provide useful information such as discolouration on bearings’ raceways, cages and balls, that itself may be the result of increased vibration, leading to wear.
Ultrasonic senses
New forms of technology-reliant condition monitoring have made use of this, says Tweedy: the human ear has been replaced by ultrasonic devices; the human eye by thermography which looks for heat that can be caused by friction. In the case of rotational equipment, and bearings in particular, ultrasonic is ideal, given that these parts often produce ultrahigh frequency sounds when vibrating. And where access is an issue, thermography is the better solution, he asserts.
“Analysing machinery for vibration is becoming increasingly popular. We are increasingly finding that maintenance related tenders have vibration analysis stipulated within them,” explains Tweedy.
“There’s a very good reason for this: vibration analysis allows early detection of wear, fatigue and failure in rotating machinery. That’s because vibration generally highlights an issue discovered by higher readings and particular frequencies, mostly as the result of wear and tear but also as a consequence of poor maintenance practices. Vibration builds and leads to equipment failure.”
Identifying potential problems and a predicted time to failure enables advance ordering of replacement parts and scheduled proactive maintenance, reducing unexpected downtime.
While many firms opt for in-house analysis, using trained consultants provides a more sophisticated level of maintenance, insists Tweedy.
“Condition monitoring in all its forms has seen a significant uptake over the past decade, with vibration monitoring and analysis gaining greater traction. Manufacturing, engineering and processing sites are seeing genuine benefits and in many instances vibration analysis is becoming business critical. Some insurance companies are even insisting on vibration analysis for safety, as we have regularly seen when it comes to electrical panels.”