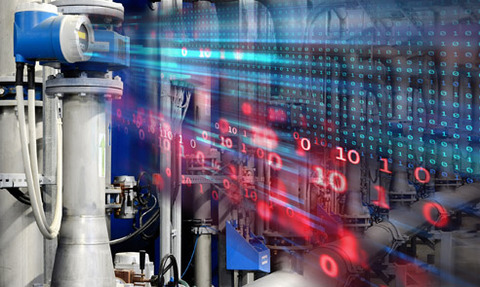
Flow measurement is attaining new depths of understanding and usefulness in the age of Big Data, agile performance and servitisation.
Former chief strategy officer of Salesforce and co-author of Subscribed: Why the Subscription Model will be your company’s future, Tien Tzuo suggests that the current industrial landscape is on the verge of a transformational change that parallels two earlier revolutions in the world of business.
At the start of the 19th century, came the efficient division of labour heralded previously by Adam Smith; in the early 20th century, the assembly line pioneered by Henry Ford.
Fast forward to the present day and the seismic change is provided by the shift from providing goods to providing services, exemplified by those modern giants such as Amazon and Spotify.
That model is expanding, not so gradually, into other sectors such as transportation (Uber) and food (Deliveroo), a fact underlined by the rapid increase in investments by financial investors – a process certain to continue after the economic effects of the Coronavirus epidemic subsides. And it is within industry we can expect to see this develop greatest, predicts Tzuo and his fellow author Gabe Weisert.
Companies working within the control and instrumentation field are wise to this, encouraged by the raised user expectations created by Industrial Internet of Things solutions. Among those early adopters Endress+Hauser has been a significant presence.
We go well beyond the established test methods within this program by scrutinising the functionality of complete system architectures in a lab environment
Jörg Reinkensmeier, marketing manager, Endress+Hauser
Its Open Integration partner programme has brought together 13 manufacturers who jointly aim to achieve the streamlined integration of their products.
These include suppliers of control technology, fieldbus infrastructures, measurement technology or actuator technology, namely AUMA Riester, Bu?rkert, Festo, Flowserve, Hima Paul Hildebrandt, Honeywell Process Solutions, Mitsubishi Electric, Pepperl+Fuchs, Phoenix Contact, Rockwell Automation, Schneider Electric, Turck and most recently Softing Industrial Automation.
“We go well beyond the established test methods within this program by scrutinising the functionality of complete system architectures in a lab environment,” explains Endress+Hauser marketing manager Jörg Reinkensmeier. “We do that for specific fields of application or customer solutions. After successful completion of the tests, the so-called reference topologies are published in the form of mutual recommendations.”
A key bonus of Softing’s involvement was its expertise in the area of data exchange. With connectivity playing a growing role in IIoT solutions, this brings an added benefit in addition to the effective networking of process control technologies.
Improved data capture translates into advances in the measurement of process variables within industrial production. Not surprisingly, TÜV SÜD’s National Engineering Laboratory (TÜV SÜD NEL) has been at the forefront of developments focused on flow measurement.
The use of algorithms to process data and then forecast trends has become ever more effective thanks to the increased sophistication of data-driven models revealing hidden trends, patterns and interrelationships in data that provide substantial insights into unseen conditions, says the company.
Where flow measurement is concerned, many different metering technologies are employed, such as Coriolis flowmeters and ultrasonic flowmeters (USMs). These flow meters come equipped with digital transmitters, says Dr Yanfeng Liang [pictured], mathematician at TÜV SÜD NEL.
These are capable of outputting a large amount of diagnostic data containing potentially valuable information regarding the performance and health of a meter within a variety of operational environments.
This can prove especially useful when identifying the specific reason for drifts in diagnostic variables, for which there may be a number of potential causes.
Many to few
“Any problems such as the presence of an unwanted second fluid phase, improper installation or particle deposition such as wax are usually manifested as drifts from baseline values. Very often, simple observations in the drifts seen in the diagnostic variables are insufficient to differentiate between errors as some can induce the same drifts and trend patterns, leaving end-users questioning which type of error is responsible for causing the observed diagnostic data drifts,” she explains.
“Therefore, delay in pinpointing the exact cause of the error will, no doubt, delay the rectification process as well as resulting in higher operating and maintenance costs.” Moreover, a drift seen in one variable does not necessarily indicate there is an issue in relation to that variable. One variable can have a ‘one to many’ relationship, cautions Liang: “Failing to take into consideration the correlations between variables will limit our understanding.
“However, attempting to determine the correlations of all variables ‘by eye’, without the use of advanced modelling techniques, becomes very challenging and inefficient, especially in flow measurement where we may be handling over 30 different digital process variables.”
Thanks to the scale and longevity of the TÜV SÜD NEL operation, it boasts a considerable back catalogue of historical data stretching back to 2003.
“As the holders of the UK’s Standard for Flow and Density Measurement, TÜV SÜD National Engineering Laboratory has gathered and stored a large volume of historical data for different flowmeters under different operating conditions,” explains Liang.
“Over the past two years, data-driven models have been constructed by our newly formed Digital Services team. The models are based on historical data and have revealed hidden trends, patterns and interrelationships which have in turn provided useful insights to help better understand their diagnostic variables and ultimately enable the creation of condition-based monitoring systems.”
Failing to take into consideration the correlations between variables will limit our understanding
Dr Yanfeng Liang, mathematician, TÜV SÜD NEL
Furthermore, she states, future predictions can be made from data-driven models which can significantly improve the decision-making process. The benefits can be illustrated with the case of a predictive model for multiphase data. The presence of a second phase, such as gas in an ultrasonic flowmeter – in which liquid measures the velocity of a fluid with ultrasound to calculate volume flow – can adversely affect performance and thus the reliability of its outputs. The degree of impact is dependent on the amount of second phase fluid present within the flow, comments Liang.
Research was conducted to analyse the effect of having different percentages of gas present within the flow on the performance of the USM. Nine sets of data (A to I) were gathered from the flowmeter with gas volume fractions (GVF) of 0.0%, 0.1%, 0.2, 0.5%, 1%, 1.5%, 2%, 5% and 10%.
Based on the data obtained, highly predictive models were constructed which enabled the prediction of the amount of gas present within the flow, based entirely on the correlations and trends observed from diagnostic variables.
“For example, based on the drifts in variables shown in Data B, the model predicted that those data were gathered when the flow meter had 0.1% of GVF with a probability of 0.9061,” outlines Liang.
“Similarly, for Data A, the model predicted with a high probability of 0.9994 that those data represent a normal operation condition.”
The predictive results highlight the datadriven models’ potential for flow measurement, she says, given that the precision in identifying the cause of drifts “will significantly improve end-users’ fault diagnosing processes.”